Variational approach to the analysis of massive
data arrays
Visual images, signals, symbolic sequences, as well as sets and
successions o f images, signals or symbolic sequences, these all
are glowing examples of massive ordered data sets. A bulk of data analysis
problems for such a kind of data lend themselves to mathematical formulation
as variational (optimization) problems with the so-called separable
objective functions, i.e. those ones which consist of additive constituents
of only one or two arguments. The aim of the research is constructing
effective generalized data analysis algorithms on the basis of decomposition
of the original optimization problem into a succession of intervening
problems with tree-like and chain-like separability of partial objective
functions. The generalized data analysis procedure is based on an extension
of the classical dynamic programming procedure onto the case of tree-like
adjacency of goal variables.
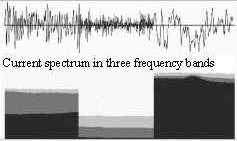 |
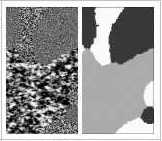 |
Edge-preserving time-frequency analysis of signals |
Segmentation of texture images |
Robust forming of feature spaces and decision
rules for the recognition of signals of different length
The natural space of the initial signal representation is that formed
by succession of the signal’s samples along the discrete axis of the
respective variable, primarily, time. Forming decision rules of signal
recognition is concerned with two fundamental barriers. First, the
dimensionality of the feature space is, as a rule, individual for
each particular signal, so, there is no common space in which
a discriminant function could be sought for. Second, the
feature-space dimensionality is practically ever much greater than
the number of signals in the training set, what turns the training
problem into an ill posed one. The theoretical research is aimed at
overcoming both of these difficulties when inferring a decision rule
from a training set.
Two-dimensional representation of a training set of high-dimensional
vectors of signal samples, and the optimal linear decision rule approximately
distinguishing between two slightly intersecting classes of signals.